Cyber-physical Systems

While wireless communication offers unprecedented flexibility in sharing data between systems, which increases collective information and allows collaborative action, it is, in comparison to wired communication, less reliable, and its bandwidth is limited. For example, if all autonomous vehicles in a big city use the same wireless network and communicate simultaneously, the whole network may break down, impeding communication.
Further, for many application examples of cyber-physical systems, such as drones, we need to do computations on lightweight devices, which limits their computational power. This is particularly challenging for machine learning algorithms, which are often demanding in terms of computations. Still, machine learning algorithms are an essential asset of cyber-physical systems. They are essential, especially because cyber-physical systems are supposed to act autonomously in the real world, and not all situations they may encounter can be anticipated at design time. Machine learning methods can bring the required flexibility and adaptability for systems to work safely in unseen situations.
The cyber-physical systems group addresses these challenges by co-designing control, machine learning, and communication, both through theoretical advances and practical experiments.
The cyber-physical systems group is led by assistant professor Dominik Baumann.
Group members
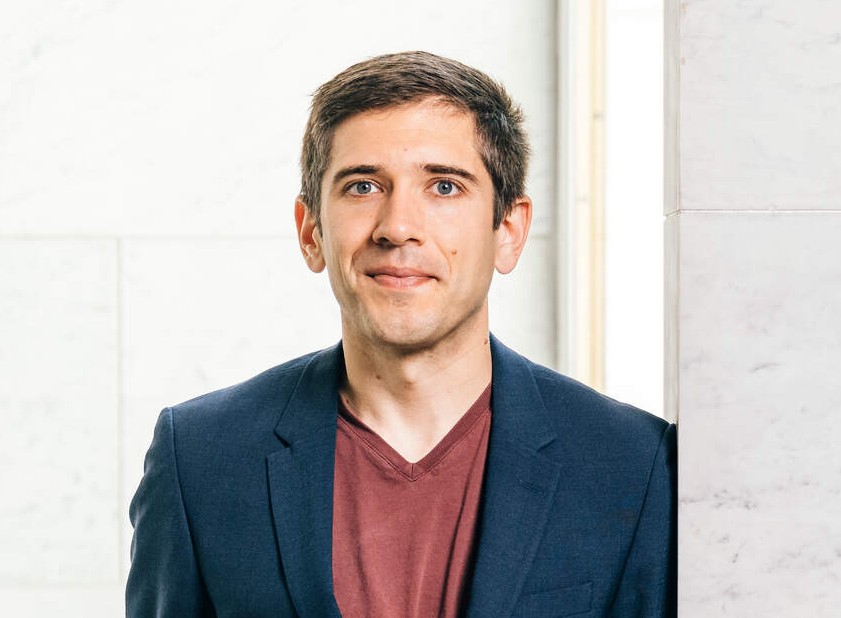