Nonlinear Systems and Control
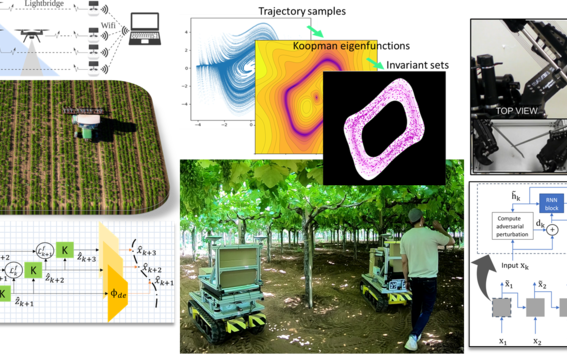
Autonomous systems are becoming ubiquitous - we may interact with delivery vehicles, self-driving cars, medical robots, or less tangible but equally pervasive AI agents like virtual assistants and recommendation systems. Designing and controlling these systems to achieve intelligent yet safe behaviors typically require careful analysis of the system architecture and dynamical representation, the type of feedback information available, actuation capabilities, model uncertainties, and other highly problem-specific considerations. Yet there is an allure of empirical machine learning-based approaches that can handle broad ranges of decisions and control tasks reasonably well.
The research interest of the Nonlinear Systems and Control group lies in the intersection of Control theory and Machine Learning: We aim to synergistically combine tools from nonlinear systems, stability theory, robust & optimal control along with machine learning and optimization techniques, to create systems with provable guarantees on safety and performance.
Our impact areas include medical and rehabilitation robotics, precision agriculture, and multi-agent systems with human-in-the-loop, among others. Besides applications in cyber-physical and robotic systems with varying levels of autonomy, the same principles and tools can be applied towards robustification of Deep Neural Network architectures and training algorithms, when the problem is examined from a dynamical systems perspective. Our group continues to explore this direction, with a special focus on Recurrent Neural Nets.
The Nonlinear Systems and Control group is led by Assistant Professor Shankar A. Deka.
Application link: Click here
- Published:
- Updated: