Bo Zhao believes machine learning systems will become like dictionaries – everyday tools we all use to solve problems

What are you researching and why?
My research focuses on efficient data-intensive systems that translate data into value for decision making in various business, science and engineering scenarios. The challenge here is to design the software systems to manage machine learning (ML) models and heterogeneous data at a very large scale. For instance, training GPT-4, the ML model behind the popular chatbot ChatGPT, requires the distribution of the model and data across tens of thousands of special hardware, graphics processing units (GPU). As a result, it becomes difficult or even impossible for common users and small and medium enterprises to apply modern ML systems in their actual business. My research aims to help them by building flexible, customizable, and distributed end-to-end ML systems.
How did you become a professor or researcher?
I can start from my name, Bo, which in Chinese means PhD – so that alone means I had to go into research!
Actually, it is my personality: I enjoy the freedom to explore different things. During my doctoral studies, I did my industry internship at Amazon Web Services with excellent compensation and sufficient resources. But I had to do whatever the manager assigned to me. In contrast, being a professor provides me the privilege, or the freedom, to pursue whatever I want.
More importantly, I really like interacting with students. On the one hand, conveying my research clearly to them helps me reflect on my own work – why did I do this, what is its impact. On the other hand, students are able to think outside of the box, which injects new ideas into my own research. And the most important thing is that I enjoy witnessing students improve themselves.
What is the high point of your career so far?
I would say the highpoint is witnessing the impact of my research in real-world settings. During my PhD, I built a data stream processing engine from scratch and published several top-tier papers. In collaboration with electronic engineering experts from the National University of Singapore, the software system was successfully applied in optimized smart grid management, shifting the peak demand for electric power companies to avoid initiating extra generators in power plants. This really gave me the feeling that I’m contributing to the world.
In the same vein, during my postdoc I built a distributed reinforcement learning system which has been integrated into Huawei’s industry-level ML framework with commercial customers in finance, healthcare and mining industry.
What do you expect from the future?
You can of course never predict the future, but I would expect machine learning systems to become more user-friendly and more flexible in scalability. First, ML systems may become a basic toolset for everyone. Ideally, users will be able to leverage an ML system like a dictionary for the English language, to solve their own problems. The hype is not only in the computer system itself but also in what value the system can bring to the real world. Second, ML systems will have the flexibility to be deployed in the most powerful quantum computers for performance and in resource-constrained devices such as our cell phones for energy efficiency and privacy concerns. Then, users can choose the most appropriate ML solutions for their own use from a wide spectrum. That would be great.
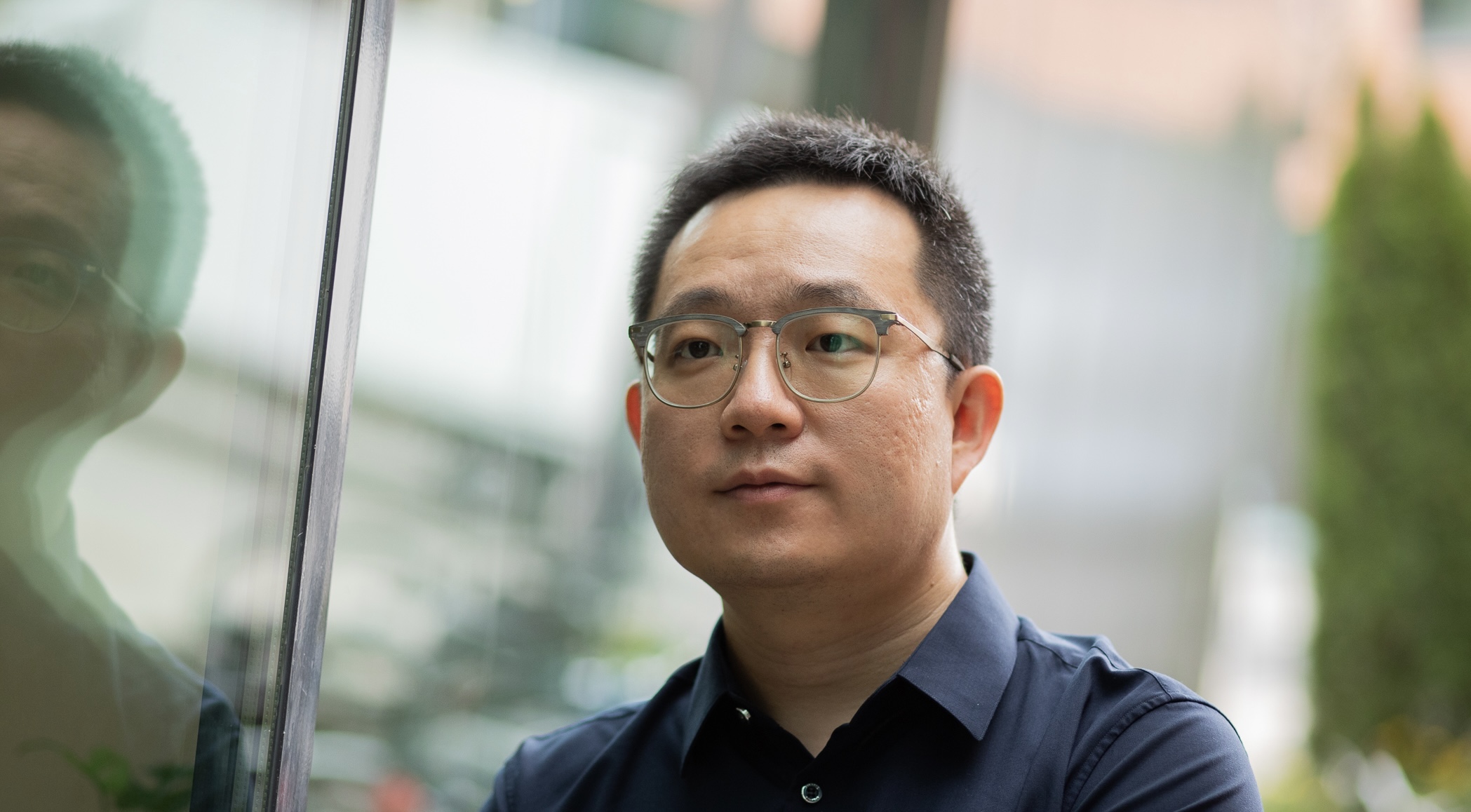
- Published:
- Updated:
Read more news

In low-hierarchy organisations, even key policy issues are discussed in Slack
In a recent study, Aalto University alumn Lauri Pietinalho, a visiting scholar at New York University's Stern School of Business, and Frank Martela, an assistant professor at Aalto University, investigated how low-hierarchy organisations deal with shared policies in confrontational situations and how authority functions within them.
Getting bacteria into line
Physicists use magnetic fields to manipulate bacterial behaviour
Nordic researchers develop predictive model for cross-border COVID spread
The uniquely multinational and cross-disciplinary research was made possible by transparent data-sharing between Nordic countries.